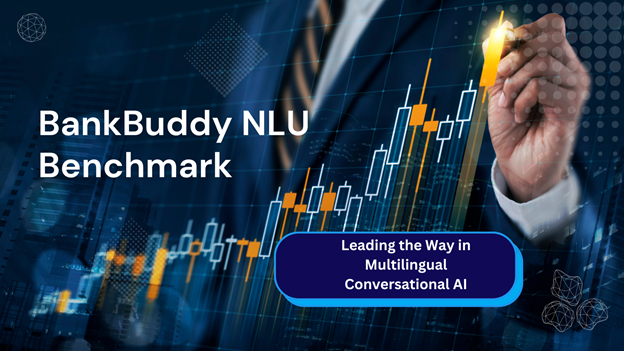
BankBuddy NLU Benchmark: Leading the Way in Multilingual Conversational AI
The field of Conversational AI has witnessed significant advancements, with Natural Language Understanding (NLU) playing a pivotal role in enabling machines to comprehend and respond to human language. As part of our commitment to delivering cutting-edge NLU solutions, we conducted a benchmarking study to evaluate the performance of BankBuddy NLU, our proprietary NLU model, against industry-leading platforms such as Rasa, Google Dialogflow, and Microsoft Azure Cognitive Service for Language. In this blog post, we present the findings of our comprehensive benchmarking report, showcasing the power and potential of BankBuddy NLU in the multilingual conversational landscape.
Dataset Selection: MASSIVE 1.1
To ensure a robust evaluation, we selected the MASSIVE 1.1 dataset, a multilingual benchmarking resource containing over one million utterances in 52 typologically-diverse languages. This dataset provides annotations for intent prediction and slot annotation tasks, making it an ideal choice for evaluating the performance of NLU models. The MASSIVE dataset is derived from the SLURP dataset, which encompasses general Intelligent Voice Assistant interactions.
Methodology: Overview of the Benchmarking Process
Our benchmarking process followed a well-defined methodology to ensure fairness and consistency across the evaluated NLU models. The key steps involved were:
1. Dataset Preprocessing: We cleaned and preprocessed the MASSIVE dataset, converting it into a suitable format for training and testing the NLU models. This step ensured compatibility with the specific requirements of each platform.
2. Model Selection: To provide a comprehensive evaluation, we selected four prominent NLU platforms for benchmarking: Rasa, Google Dialogflow, Microsoft Azure Cognitive Service for Language, and BankBuddy NLU. These platforms represent a diverse range of industry-standard solutions.
3. Model Training: We trained each NLU model using their respective algorithms, user interfaces, or APIs. The training process involved optimizing model parameters and minimizing prediction errors using the preprocessed dataset.
4. Model Testing: Once trained, we evaluated the performance of each NLU model on a separate test dataset. This dataset comprised unseen data, ensuring the models' ability to generalize to new inputs consistently.
5. Evaluation Metrics: Performance evaluation was carried out using well-established metrics, including accuracy, precision, recall, and F1 score. These metrics provided a comprehensive understanding of each model's strengths and weaknesses.
Results Analysis: BankBuddy NLU Benchmark Overview
The benchmarking results revealed the exceptional performance of BankBuddy NLU across multiple key dimensions, solidifying its position as a top-tier conversational AI solution. Let's delve into the performance analysis:
Intent Accuracy Results:
BankBuddy NLU consistently outperformed Rasa, Google Dialogflow, and Microsoft Azure Cognitive Service for Language in intent classification across all 11 tested languages. The following table presents the intent accuracy results across the 11 languages:
Language | Rasa | Google Dialogflow | Azure Cognitive Service For Language |
BankBuddy |
---|---|---|---|---|
Afrikaans | 80.30% | 83.39% | 84.63% | 87.12% |
Arabic | 75.29% | Not supported | 80.40% | 81.88% |
Bengali | 78.61% | 79.22% | 83.89% | 84.26% |
Chinese | 78.75% | 81.61% | 84.70% | 86.45% |
English | 80.83% | 83.62% | 87.83% | 89.14% |
French | 80.73% | 83.19% | 85.74% | 87.76% |
Hindi* | 78.18% | 80.53% | 85.14% | 84.40% |
Indonesian | 80.36% | 83.05% | 85.71% | 86.72% |
Spanish | 80.16% | 81.64% | 85.71% | 86.55% |
Tamil* | 77.10% | 76.13% | 83.19% | 82.78% |
Urdu | 78.61% | Not supported | 83.25% | 85.41% |
Average | 78.99% | 81.38% | 84.56% | 85.68% |
*Although, Google Dialogflow and Azure Cognitive Service For Language claim to be multilingual in nature, their multilingual capabilities are limited as their multilingual NLU depends on individual models trained for each language. Whereas, in the case of Rasa and BankBuddy NLU, we are able to get predictions in all 11 languages from a single multilingual model. Hence, although on paper, the results of Google Dialogflow and Azure Cognitive Service For Language might look impressive, their multilingual use case is limited as the API call to Google Dialogflow and Azure Cognitive Service For Language require the language of the text to be passed in the body of the API call. Hence, to ensure the fairness of the benchmark, we also train individual models for Hindi and Tamil on BankBuddy NLU and obtain an Intent Accuracy of 85.44% in Hindi and 83.36% in Tamil.
Entity Recognition Results:
BankBuddy NLU showcased remarkable accuracy in entity recognition, achieving higher strict and partial F1 scores compared to Rasa. However, due to tokenization limitations in Rasa for certain languages like Chinese, separate models were trained for a fair comparison. The following table presents the entity recognition results:
Language | Rasa | BankBuddy | ||
---|---|---|---|---|
Strict F1 | Partial F1 | Strict F1 | Partial F1 | |
Afrikaans | 66.85% | 72.52% | 77.64% | 82.31% |
Arabic | 70.40% | 76.76% | 75.55% | 80.96% |
Bengali | 70.69% | 76.11% | 75.33% | 80.22% |
Chinese* | 63.26% | 72.78% | 68.22% | 78.49% |
English | 63.42% | 69.02% | 81.56% | 85.49% |
French | 46.79% | 54.22% | 74.38% | 79.07% |
Hindi | 64.46% | 71.48% | 73.22% | 78.94% |
Indonesian | 66.16% | 73.77% | 75.94% | 81.45% |
Spanish | 60.06% | 67.24% | 73.45% | 78.61% |
Tamil | 66.76% | 73.31% | 73.84% | 79.48% |
Urdu | 63.56% | 70.04% | 71.47% | 77.91% |
Average | 63.86% | 70.66% | 74.60% | 80.27% |
*Rasa’s WhitespaceTokenizer only supports whitespace tokenizable languages. Tokenization is the identification of Linguistically Meaningful Units (LMU) from the surface text. Thus, satisfactory results cannot be achieved for Chinese, which is not a whitespace tokenizable language using the multilingual configuration of Rasa for NER. Thus, for the fairness of the benchmark. We train a separate model for Rasa and BankBuddy NLU for Chinese NER. The results of the same are established in the table.
Overview of the Multilingual Potential of BankBuddy NLU
One of the standout features of BankBuddy NLU is its robust multilingual capabilities. While other platforms may claim multilingual support, their capabilities are often limited to individual models trained for each language. BankBuddy NLU, on the other hand, delivers true multilingual functionality with a single, versatile model capable of handling all 11 languages tested in this benchmark. This provides a significant advantage in terms of ease of use, scalability, and cost-effectiveness.
In addition, we ensured a fair comparison by training separate models for Hindi and Tamil on BankBuddy NLU, achieving impressive intent accuracies of 85.44% in Hindi and 83.36% in Tamil. This highlights BankBuddy NLU's ability to handle specific languages with remarkable precision.
Conclusion: Multilingual Performance of BankBuddy NLU
The benchmarking study conclusively demonstrates that BankBuddy NLU sets new benchmarks in multilingual conversational AI. With superior intent accuracy and entity recognition capabilities, BankBuddy NLU outshines Rasa, Google Dialogflow, and Microsoft Azure Cognitive Service for Language across the tested languages. Its true multilingual support and scalability make it an ideal choice for businesses and developers seeking a robust NLU solution for their conversational AI needs.
As we continue to refine and enhance BankBuddy NLU, we remain committed to driving innovation in Conversational AI and delivering state-of-the-art solutions that empower organizations worldwide.
To learn more about BankBuddy NLU and its applications, visit our website or get in touch with our team today.