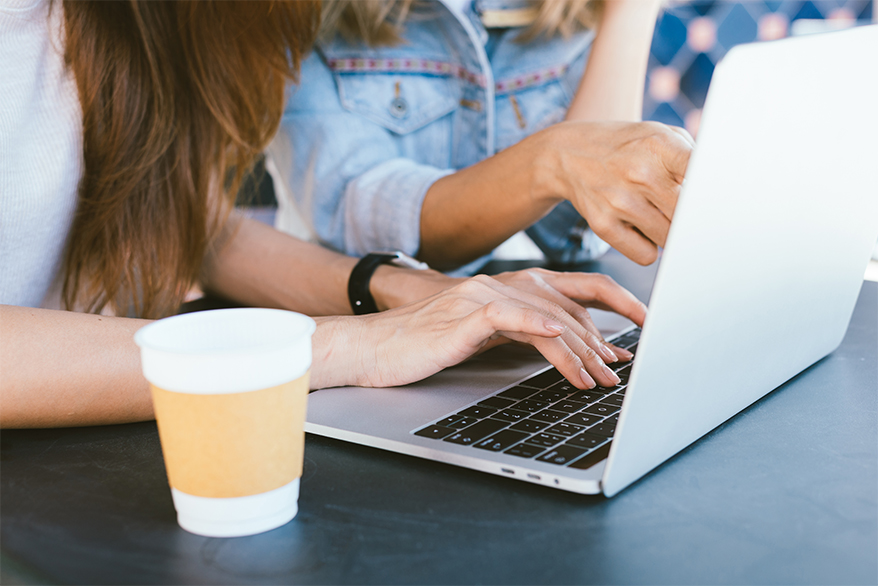
Domain Specific Ontologies – Relevance & Context in Applied AI
By Sivaramakrishnan R Guruvayur
Chief Data & ML Scientist – BankBuddy.ai
Research scholar, Jain University
Email : shibu@bankbuddy.ai
What is domain ontology?
Ontologies find use in the Semantic Web, Artificial Intelligence, Systems Engineering, Biomedical Informatics, Software Engineering, Enterprise Bookmarking, Library Science, and Information Architecture in a type of representation of knowledge about the domain or a part of the domain.
There are 4 groups of ontologies: static, dynamic, social and intentional.
- A static ontology elaborates items in existence; their attributes and the relationship with them.
- Dynamic ontology explains the domain as states and their transitions including processes.
- Social ontology describes social scenarios, permanent structures in an organisation or changing networks of independencies and alliances.
- Intentional ontology includes the domain of agents, things wanted, believed in, proved, disproved, and discussed about.
Creating a domain ontology is also key to defining and using a framework of enterprise architecture. Therefore, the first stage of creating an effective system for representation of knowledge and vocabulary is an effective ontological study of the domain. A weak analysis will result in knowledge bases that are incoherent.
For building a language of knowledge representation based on analysis, an association of terms along with ontological concepts and relations, and creation of a syntax for knowledge encoding pertaining to the concepts and relations is necessary. This language of knowledge representation can be shared with those having similar requirements for representing knowledge in that domain, hence negating the requirement for repeating the process of knowledge analysis. Ontology sharing can hence create the basis for domain specific information-representing languages. When compared with the earlier generation of such languages (say, KL-1), these languages have rich content besides having a many terms embodying complex constituent of the domain.
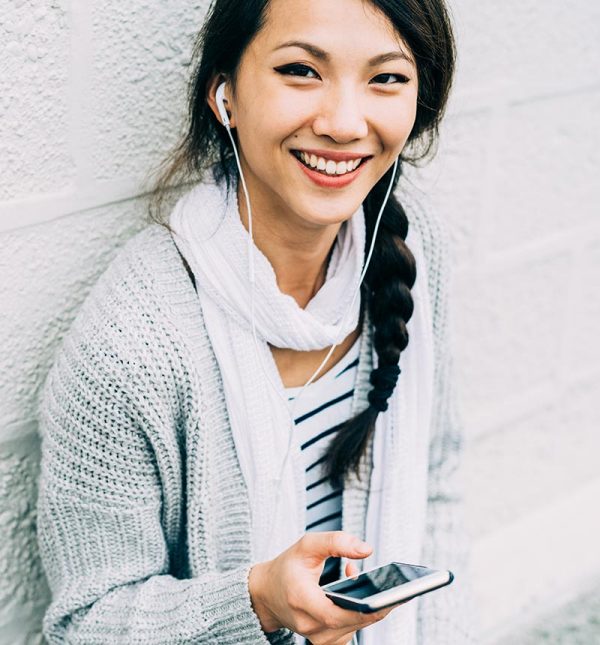
Why create domain specific ontologies ? – “Context is the King”
Domain specific ontologies are created for the following purposes:
- Sharing common understanding of structures of knowledge among software agents or users
- Enabling reuse of domain knowledge for consumption of upstream and downstream AI applications
- Making explicit domain assumptions – Connected data
- Separating operational and domain knowledge
- Analysing knowledge of the domain
Challenges in Building Domain Specific Ontologies
Following are the challenges in building domain specific ontologies:
- Insufficient coverage for limited domains: Typically, the coverage for domains having lesser web presence is lesser than that of domains that are more popular.
- Relational identification: Generally, the extraction relations are not spelled out in advance. Hence, for domain specific ontologies, identifying relations needs deep domain expertise.
- Resolving entities: The issue of identification and grouping/linking various manifestations/occurrences of the same real-world item is a difficult task.
- Disambiguation of Entities: A phrase or word may imply more than one entity. Entity disambiguation involves association of the phrase/word with the most relevant entity.
- Problem of temporal knowledge base: There are facts which vary with time, hence mapping the phrase/word with the relevant entity can be an issue.
- Extracting values: Ontologies are generally represented in the form of triples, i.e.,
. The system is required to learn all possible formats of entity/relationship to enrich the ontology. - Confidence of facts: There are many disputable facts which depend on the source of information. Hence, it is often difficult to locate a unique entity if there is a conflict.
Connected Data – Ontologies and Knowledge Graphs
As mentioned earlier, an ontology can be defined as an explicit, formal specification relating to a shared conceptualization based on a high expressiveness of semantics needed for higher complexity. Representations of Ontology permit modelling of knowledge semantically, and are thus widely utilised as artificial intelligence application knowledge base for knowledge-based systems. Applying an ontology as a knowledge graph allows validating semantic relations and deriving conclusions from available facts for reasoning.
Ontologies, essentially, are not different from knowledge graphs, though they are occasionally classified erroneously in similar level of database schemas. Ontologies, in fact, comprise of not only properties and classes, but also have instances such as the ontology population. Putting it differently, size sometimes is mentioned as a key feature of knowledge graphs (KGs), hence a KG can be thought of as large ontology. But then, it has been pointed out that KGs are, in a way, superior to an ontology and possess additional characteristics. Hence, the difference between KGs and ontologies can be seen either in a quantitative manner as a large ontology, or of having extended needs with a built-in tool which permits derivation of new knowledge. The latter interpretation implies that a KG is a knowledge-based system which has a reasoning engine and a knowledge base. Looking at current automatically generated KGs, we could identify the next important feature: collecting, extracting, and integrating inputs from external sources stretches an essentially knowledge-based system with the idea of system integration.
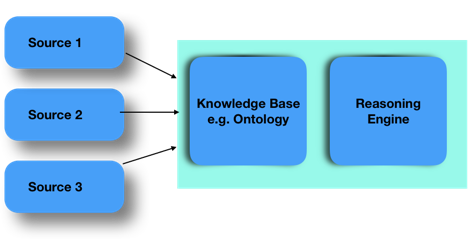
Machine Learning Methods and Ontologies
Machine Learning is a discipline that can contribute in a major way in improving ontology creation and learning. A classical explanation of machine learning (ML) is as follows: an experience E teaches the system in accordance with a performance indicator P if it enhances its performance measured by P after going through the experience E. ML, traditionally, is based on developing inductive pattern extracting methods from the provided data. It goes beyond statistical research for model building and works with much more complex algorithms to obtain precise and larger models for the data, which may not be comprehensible to humans any more. ML finds wide use in areas involving prediction, adaptation, and pattern recognition and extraction.
The relation between ontologies and types of ML algorithms used for learning of these ontologies is presented in
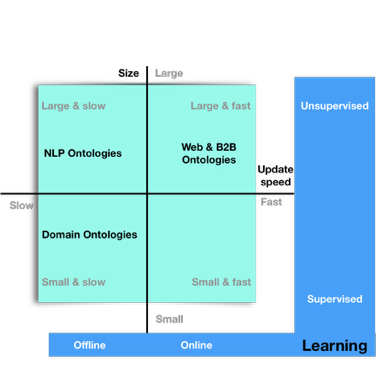
Fig 2: Ontology & ML type
Table 1 presents the comparison of the kinds of ML algorithms with types of ontologies. It is seen that each kind of ontology can be learned by a specific ML technique.
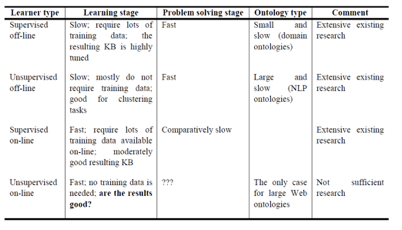
In the next post we will discuss in details about how different ML algorithms can be used to create domain ontology for specific use cases and business challenges.
To foster superior and automated customer interactions, get in touch with us at https://bankbuddy.ai/